Visit our REU Vir(tu)al Conference 2021 to learn about what we worked on during the summer. There has been much progress since.
Quasistationary Distributions for the Invasion and Voter model
Clay Allard (University of Utah), Shrikant Chand (New York University) and Julia Shapiro (University at Buffalo)
This project continues from where we left off in MCREU `20. We discussed and developed better methods for simulations and used them to formulate a conjecture for the limiting QSD for the model, which eventually led us to prove a limit theorem for the QSD for the model. Our work involved multiple techniques and the main argument used in our proof is an application to Stein’s method to our model.
The simple Voter and Invasion models on complete bipartite graphs and our work on them (MCREU `19 on Voter) appear to demonstrate in precise mathematical terms long-term dynamics of opinions under two distinct social norms:
- In the voter model the agents “listen”, and accept other opinions. Here we observed that the system, conditioned on not reaching a consensus will be very close to consensus, with a heavy-tailed distributed number of dissents from the opinion.
- In the invasion model, the agents “force” their opinion. Here we observed that the system, conditioned on not reaching a consensus will be very disordered: the density of each of the two possible opinions (“yes” or “no”) will be uniformly distributed and rarely changed among those with fewer connections, while the opinions of those with many connections, conditioned on the density of “yes” opinions in the former group to be equal to some value p, will be independent and identically distributed with probability x of being yes for each.
- Manuscript arXiv:2204.10287
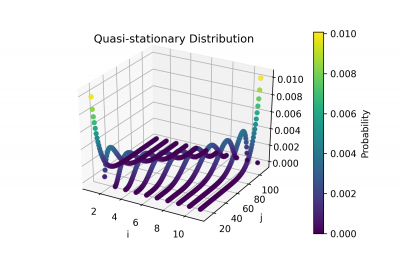
-
A talk given by Dr. Ben-Ari on this (including sketch of the proof of our main result) and related work at a Symposium on Stochastic Hybrid Systems and Applications, UConn (November 2021).
- Video of talk given by participants in the REU Conference in the summer (before project was completed)
Ballistic Deposition Model
Connor Bass (Macalester College), Na’ama Nevo (Colorado College), Caitlyn Powell (U of Alabama)
The Ballistic Deposition Model is a Markov Chain on the set of “height” functions on vertices of a finite graph. Initially, each vertex is assigned some height (think of a height of a building on the vertex), and each unit of time a vertex is uniformly sampled and the height is updated to one plus the maximum among the heights all neighbors and the vertex itself. The height at each vertex grows asymptotically linearly with a deterministic speed. In this project we tried to improve existing upper and lower bounds on the speed on cycle graphs. Our work involved:
- Getting good simulations for the model to learn empirically about the dependence of the speed on the structure of the graph, and trying to find simper structures where a tight estimate for the speed is more accessible than for cycle graphs.
- Learning about subadditivity the reason for the linear growth.
- Working with stochastic domination to obtain upper bounds.
- Working with large deviations to obtain lower bound.
The work done will be presented in Joint Mathematical Meetings in January 2022.
Coalescing and Annihilating Random Walks
Chris Vairogs (University of Florida) and Daniel Zou (Reed College)
In this project we studied a particle system consisting of multiple random walkers on a cycle graph, which upon meeting in one model coalesce and in the other model annihilate. The main task was to study the time until there is only one (or less) particle remaining. Our focus was on the models in the cycle graph, where some numerical results on the expectation and variance were previously reported.
- We proved the formulas for the expectation and worked on the variance; and
- Through analysis of simulations, we obtained a conjectured exact formula for the variance, an improvement over the existing big-Oh numerical results. We are still working on a proof.
The work on this project introduced analysis based on construction of nonstandard martingales and utilizing optional stopping.